In the future, Generative AI holds immense potential to significantly enhance user experiences and bolster decision-making capabilities.
Generative AI: The Next Frontier of AI
Generative AI is a type of artificial intelligence that can create new content, such as text, images, and music. It does this by learning from existing data and then using that data to generate new outputs that are similar to the data it was trained on. According to the Worldwide Artificial Intelligence Spending Guide released by the International Data Corporation (IDC) in February 2023, India’s expenditure on AI is projected to reach $3.6 billion by 2026, exhibiting a compound annual growth rate (CAGR) of 34.3%. Generative AI, having sparked the interest of a multitude of organizations beyond just tech startups, is driving the development of large language models (LLMs) or the creation of specific use cases to meet their business demands.
AI: Evolution
Generative AI is a type of artificial intelligence that can create new content, such as text, images, or music. It is based on the idea that machines can learn to generate realistic output by analyzing large amounts of existing data.
1960s
The evolution of generative AI can be traced back to the early days of artificial intelligence when researchers began to explore the possibility of creating machines that could learn to generate new information. One of the first generative AI systems was ELIZA, a chatbot developed in the 1960s that could simulate human conversation.
1980s
In the 1980s, researchers developed new generative AI techniques based on neural networks. These techniques were more powerful than previous methods, and they allowed machines to generate more realistic output. However, they were also more computationally expensive, which limited their use.
2010s
In the 2010s, the development of deep learning led to a new wave of progress in generative AI. Deep learning is a type of machine learning that uses artificial neural networks to learn from data. It is more powerful than previous machine learning techniques, and it has allowed machines to generate increasingly realistic output.
One of the most significant advances in generative AI in recent years has been the development of generative adversarial networks (GANs). GANs are a type of deep learning model that can generate realistic images, videos, and audio. They work by pitting two neural networks against each other in a competition. One network, the generator, tries to create realistic output. The other network, the discriminator, tries to distinguish between real and fake output. As the two networks compete, they both improve, and the generator eventually becomes capable of creating highly realistic output.
GANs have been used to generate a wide range of content, including images, videos, and music. They have also been used to create new forms of art and entertainment. For example, the GAN-generated images of celebrities have been used to create deep fakes, which are videos that have been manipulated to make it appear as if someone is saying or doing something they never actually said or did.
Generative AI is a powerful tool that has the potential to revolutionize many industries. It can be used to create new products and services, improve existing products and services, and automate tasks. As generative AI continues to evolve, it is likely to have a profound impact on the world.
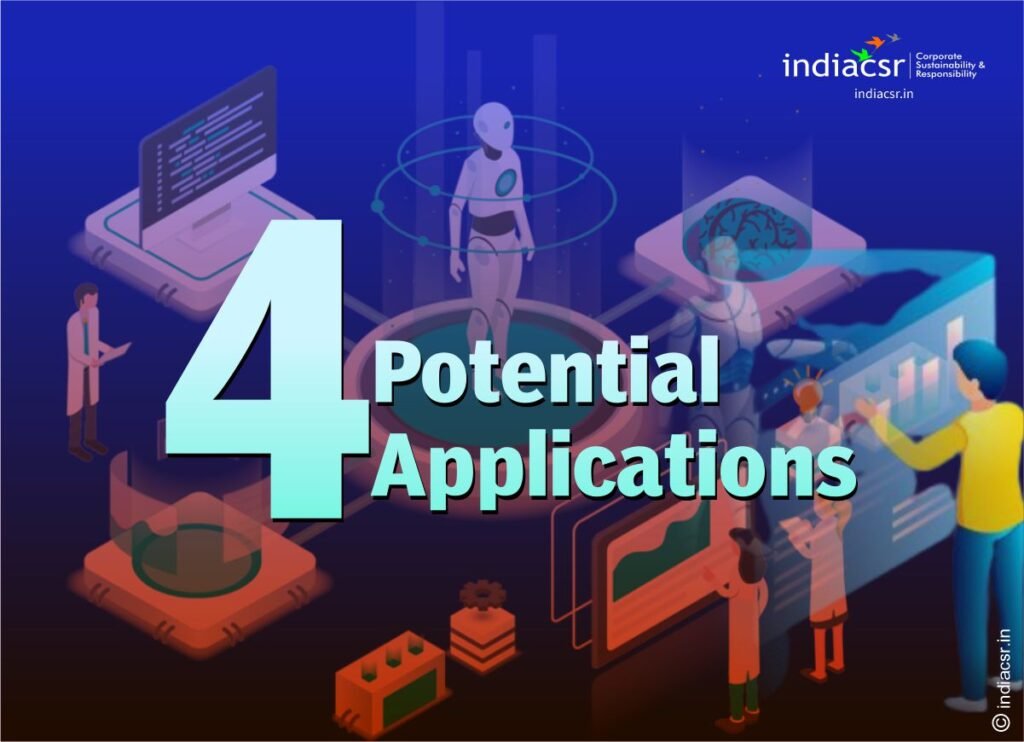
Generative AI: 4 Potential Applications
Generative AI has a wide range of potential applications, including:
Content creation: Generative AI can be used to create new content, such as articles, blog posts, and even books.
Data augmentation: Generative AI can be used to augment existing data sets, which can be helpful for training machine learning models.
Data compression: Generative AI can be used to compress data, which can save space and improve performance.
Privacy protection: Generative AI can be used to protect privacy by generating synthetic data that is indistinguishable from real data.
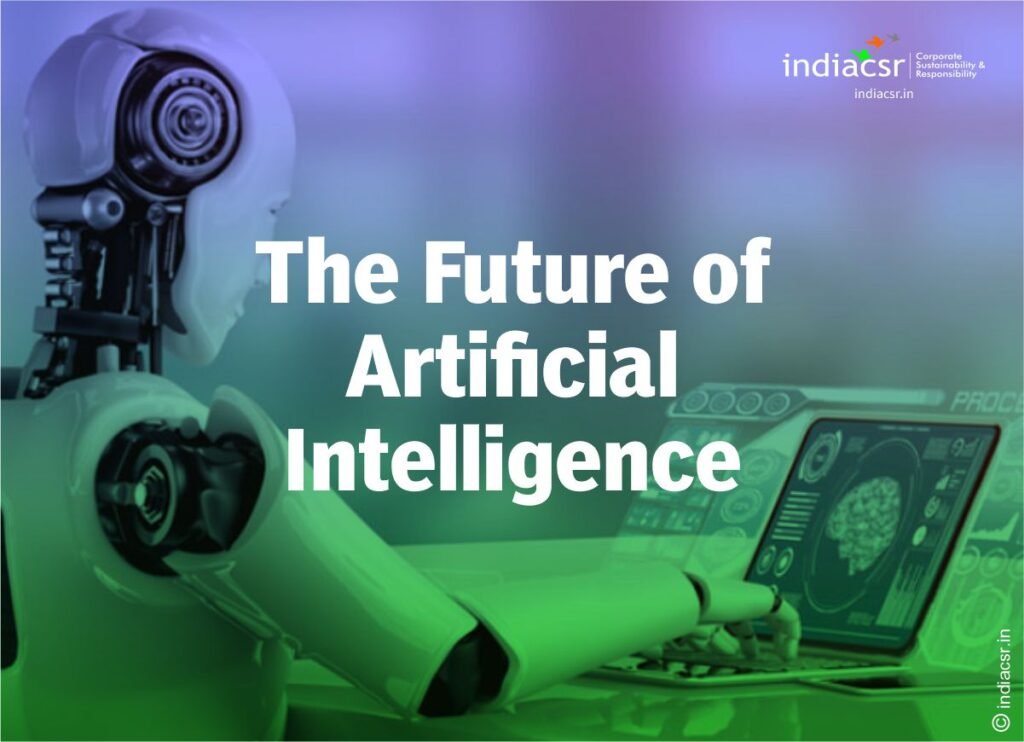
Generative AI: The Future of Artificial Intelligence
Generative AI is one of the most promising areas of artificial intelligence research. It has the potential to revolutionize a wide range of industries, including healthcare, finance, and entertainment.
In healthcare, generative AI can be used to develop new drugs and treatments, as well as to create personalized medical plans. In finance, generative AI can be used to create new investment strategies and predict market trends. In entertainment, generative AI can be used to create new movies, TV shows, and video games.
The potential applications of generative AI are endless. As the technology continues to develop, it is likely to have a profound impact on our lives.
How Generative AI Works
Generative AI works by learning from existing data. This data can be anything from text to images to audio. Once the AI has learned from the data, it can use that data to generate new outputs that are similar to the data it was trained on.
There are a number of different ways to train a generative AI model. One common approach is to use a technique called deep learning. Deep learning is a type of machine learning that uses artificial neural networks to learn from data.
Another approach to training a generative AI model is to use a technique called reinforcement learning. Reinforcement learning is a type of machine learning that allows an AI agent to learn by trial and error.
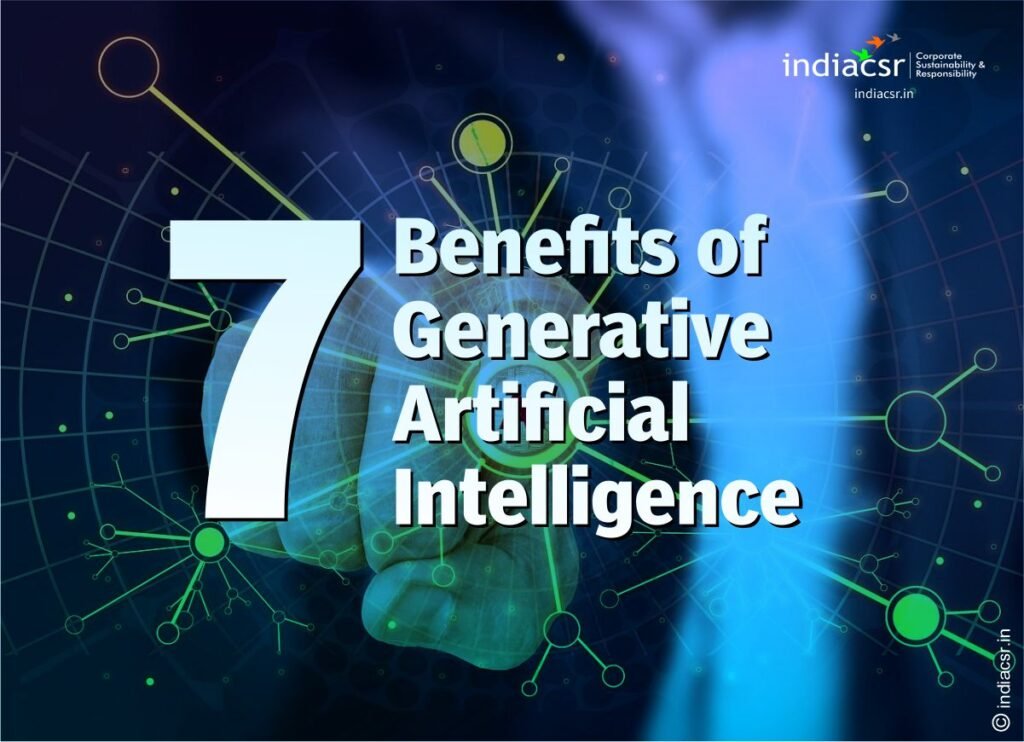
The 7 Benefits of Generative AI
Generative AI has a number of benefits. Here are some of the potential benefits, including:
- Automating tasks: Generative AI can be used to automate tasks that are currently done by humans. This can free up human workers to focus on more creative and strategic tasks.
- Fostering innovation: Generative AI can be used to create new products and services. This can help to drive economic growth and create new jobs.
- Enhancing human creativity: Generative AI can be used to help humans be more creative. This can lead to new forms of art, music, and literature.
- Increased productivity: Generative AI can automate tasks that are currently done by humans, which can free up time for humans to focus on more creative and strategic work.
- Improved quality: Generative AI can create content that is of higher quality than what humans can create. This is because generative AI can learn from a vast amount of data and then use that data to generate outputs that are optimized for a specific task.
- Reduced costs: Generative AI can reduce costs by automating tasks that are currently done by humans. This can save businesses money on labor costs.
- Increased innovation: Generative AI can help businesses to innovate by creating new products and services. This can help businesses to stay ahead of the competition.
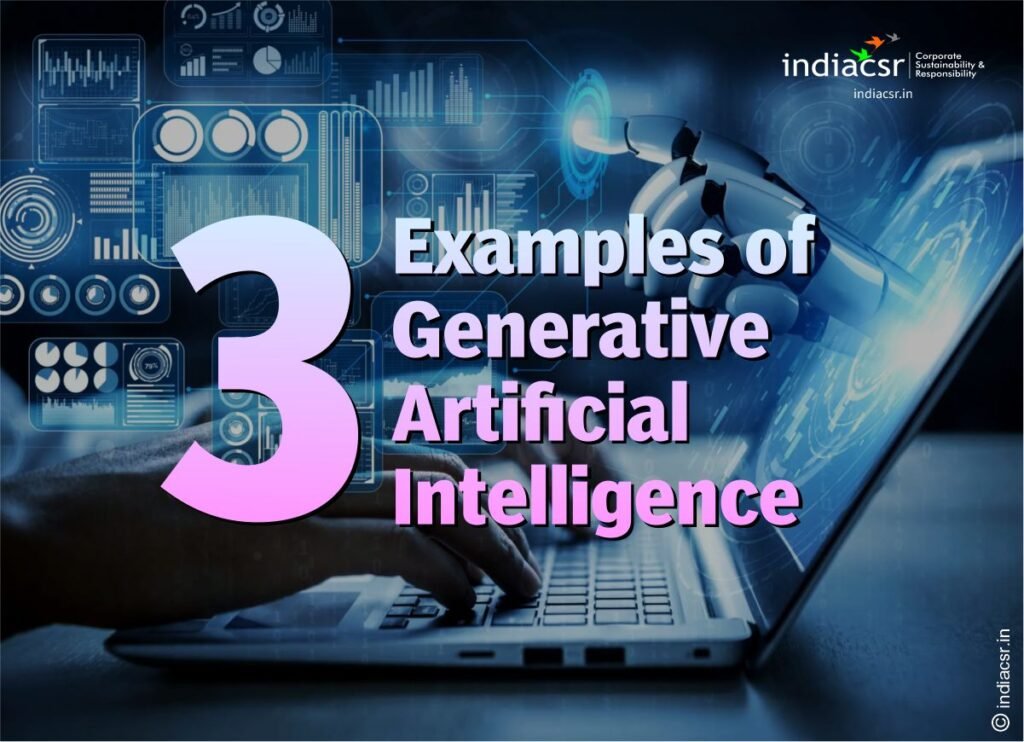
3 Examples of Generative AI
There are a number of examples of generative AI in use today. Some of the most common examples include:
- Text generation: Generative AI can be used to generate text, such as articles, blog posts, and even books. This is being used by businesses to create content for their websites and marketing materials.
- Image generation: Generative AI can be used to generate images, such as photos, paintings, and even sculptures. This is being used by businesses to create marketing materials and to generate realistic images for use in movies and video games.
- Music generation: Generative AI can be used to generate music, such as songs, soundtracks, and even entire albums. This is being used by businesses to create music for their marketing campaigns and to create new genres of music.
Trends in Generative AI
The field of generative AI is rapidly evolving. There are a number of trends that are expected to continue in the coming years, including:
- Increased use of deep learning: Deep learning is becoming the dominant approach to training generative AI models. This is because deep learning has been shown to be very effective at learning from large amounts of data.
- Increased use of reinforcement learning: Reinforcement learning is becoming increasingly popular for training generative AI models. This is because reinforcement learning allows AI agents to learn by trial and error, which can be very effective for tasks that are difficult to define or that require a lot of creativity.
- Increased use of generative AI in the real world: Generative AI is starting to be used in a wider range of applications in the real world. This is due to the increasing availability of data and the increasing sophistication of generative AI models.
What are the Top 10 AI Generative?
There are many different types of generative AI models, but some of the most popular and successful ones include:
- Generative Adversarial Networks (GANs): GANs are a type of machine learning model that can be used to generate new data that is similar to existing data. They work by training two models against each other: a generator and a discriminator. The generator is responsible for creating new data, while the discriminator is responsible for trying to distinguish between real data and generated data. As the two models train, they become better and better at their respective tasks.
- Variational Autoencoders (VAEs): VAEs are another type of machine learning model that can be used to generate new data. They work by first encoding the existing data into a latent space, and then decoding that latent space into new data. The latent space is a lower-dimensional representation of the data, which makes it easier for the model to learn the relationships between different features.
- Diffusion Models: Diffusion models are a newer type of generative AI model that has been shown to be very effective at generating realistic images. They work by gradually adding noise to an image until it reaches a desired level of realism. This process is called diffusion, and it allows the model to learn the distribution of natural images.
These are just a few of the many different types of generative AI models that are currently being developed. As AI research continues to advance, we can expect to see even more powerful and sophisticated generative AI models being developed in the future.
Here are some of the top 10 generative AI companies in 2023:
OpenAI
DeepMind
Google AI
Nvidia
OpenAI Five
DeepMind Five
Google Brain
Nvidia Clara
Amazon SageMaker
Microsoft Azure
These companies are all working on developing new and innovative generative AI models that have the potential to revolutionize a wide range of industries.
Challenges
It is important to use generative AI ethically. This means using it in a way that does not harm people or society. It also means using it in a way that is transparent and accountable.
Overall, generative AI is a powerful tool that has the potential to both benefit and harm society. It is important to be aware of the potential benefits and challenges of generative AI so that we can use it responsibly.
Here are some of the potential challenges of generative AI:
- Bias: Generative AI models can be biased, which can lead to the creation of inaccurate or misleading content.
- Privacy: Generative AI models can be used to generate realistic images and videos of people without their consent. This can be a privacy concern.
- Security: Generative AI models can be used to create deepfakes, which can be used to spread misinformation and propaganda. This can be a security concern.

The Future of Generative AI
Envisioning an era of transformation and enhancement, the future of Generative AI shines brightly, with its boundless potential to revolutionize a myriad of industries and profoundly augment the quality of our lives. It is important to use this technology responsibly and ethically so that it can be a force for good in the world.
“The success of AI is not ‘more of it’ but effective adoption with thorough understanding, planning and deployment for speed and scale. And, given its incredible benefits, it should have guard rails in place to ensure it is effective and a force for good – for both society and the economy.” Sandip Patel, IBM India Managing Director said in a recent article.
A new forecast from the International Data Corporation (IDC) Worldwide Artificial Intelligence Spending Guide shows that global spending on artificial intelligence (AI), including software, hardware, and services for AI-centric systems*, will reach $154 billion in 2023, an increase of 26.9% over the amount spent in 2022. The ongoing incorporation of AI into a wide range of products will result in a compound annual growth rate (CAGR) of 27.0% over the 2022-2026 forecast with spending on AI-centric systems expected to surpass $300 billion in 2026.
Three of the leading AI use cases in terms of spending focus on sales and customer service functions: Augmented Customer Service Agents, Sales Process Recommendation and Augmentation, and Program Advisors and Recommendation Systems. These three use cases will see investment from nearly every industry and combined will account for more than a quarter of all AI spending in 2023. The other use cases that will see the largest spending this year support a more diverse set of tasks: IT Optimization, Augmented Threat Intelligence and Prevention Systems, and Fraud Analysis and Investigation.
The two industries that will deliver the largest AI investments in 2023 and throughout the forecast are Banking and Retail. The next largest industry for AI spending will be Professional Services followed by Discrete and Process Manufacturing. Together, these five industries will account for more than half of all spending on AI-centric systems this year. The fastest growth in AI spending will come from the Media industry with a five-year CAGR of 30.2%. However, much like use case spending, only two of the industries in the Spending Guide will have CAGRs of less than 25%.
(India CSR Research)